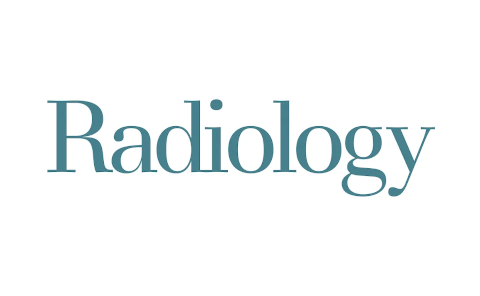
New thoughtful article in Radiology about CAD
Radiology brings an interesting article entitled “Computer-aided Diagnosis: How to Move from the Laboratory to the Clinic”, which presents a remarkably deep analysis of the current state of CAD (abstract). CAD is considered to have a great future, but a number of hard challenges need to be met. BoneXpert is featured in the article, and this “Topic of interest” post follows up on this honorable mentioning by reviewing the paper and placing BoneXpert in a wider perspective.
The authors, Bram van Ginneken, Cornelia M. Schaefer-Prokop, and Mathias Prokop from Radboud University Nijmegen Medical Centre, cleverly defines CAD in a wide sense, including detection, diagnosis as well as quantitative image analysis. The latter has brought new life to CAD in recent years – according to van Ginneken and colleagues:
Computerized quantification may hold more potential than computerized detection. When radiologists are asked to name aspects of their work that are common, time consuming, and could be automated, they usually do not mention detection but rather documentation and quantification
The authors present a detailed account of CAD for lesion detection – the most common application so far. They point to several problematic aspects:
- It is surprisingly difficult to validate CAD for detection because its performance is intricately coupled to the fairly uncontrollable behaviour of the human operator. It is much easier to evaluate the standalone performance, i.e. how well the CAD does on its own, however, this is not the intended use.
- The strict focus on detection, i.e. not allowing credibility scores to each finding, makes these system less powerful than they could be.
- FDA’s requirements to CAD are quite peculiar, as recognised also by the agency itself in 2009, but no new guidelines have been approved, so the industry is frustrated
The paper sets up four requirements to CAD (quote):
a) CAD should improve radiologists’ performance.
b) CAD should save time.
c) CAD must be seamlessly integrated into the workflow.
d) CAD should not impose liability concerns and the incremental costs should be negligible or reimbursed.
How does BoneXpert score on these requirements?
a) BoneXpert bone age gives better precision, and better accuracy as measured objectively in adult height prediction, and these improvements are clinically significant.
b) BoneXpert saves the time used for manual rating, between 1 and 15 minutes
c) With the BoneXpert standalone program, workflow integration was a major concern, but with the new PACS plugin, the result appears inside PACS as a new image in the same study.
d) BoneXpert has been shown to make fewer gross errors (defined e.g. as more than 1.5 years wrong) than manual rating in four studies. And while the cost per analysis is perhaps not negligible, BoneXpert is at least considered cost-effective: usually half of the cost is justified by the saved time, and half by the improved measurement quality.
Van Ginneken and colleagues are convinced that CAD’s importance will continue to grow, but they recognise the following obstacles for progress:
- CAD is simply very difficult to construct, because of the challenge in translating expertise into computer programs. The traditional “funnel” approach, where the CAD first extracts image features, which are then processed further into a final result, is laborious to construct, and the isolated features tend loose context. It is therefore desirable to exploit machine learning to automatically “sieve” the huge amount of available data and thereby result in an algorithm, which should also aim at a more holistic processing approach in order to mimic the intuition of human operators.
- There is a problem in measuring performance and finding clinically relevant metrics.
- The regulatory process is costly, in particular when human operators are involved.
- The integration with PACS is not easy: PACS vendors do not support an open interface which allows apps to be installed, including their own interface, with the same ease as in mobile phones.
How does BoneXpert address these challenges?
- BoneXpert is a knowledge-intensive system, containing detailed models of the shape and appearance of 15 hand bones from age 2 to adulthood. This knowledge was not programmed in the traditional way but learned from examples using a machine learning technique called active appearance models, which also provides a high degree of image understanding, so that the system knows when it is wrong. BoneXpert analyses plain X-rays, so there are large, excellent archives of data dating back to the 1950’es. Some of these data lack reliable “ground truth”, but unsupervised learning was used to learn an "intrinsic" bone age, and supervised learning was used only in the final, less complex learning steps.
- Measuring performance was solved by ”creative” use of the data: precision was measured by comparing measurements on left and right hand X-rays, and accuracy was assessed by the ability to predict adult height, an objective and clinically relevant metric.
- The intended use of BoneXpert is to replace, rather than assist, manual rating. This more ambitious goal implies absence of a human factor, and – surprisingly – it renders the regulatory path much easier, because validation can be performed as retrospective studies.
- PACS integration also becomes much easier when there is no human interaction. BoneXpert is simply installed on a arbitrary PC with network connection to the PACS. It acts as a DICOM node that one can send to, and the node returns the image to the same study. All PACS vendors support this integration mode out-of-the-box, because it is pure DICOM.
BoneXpert’s approach to manoeuver among these obstacles should encourage and inspire new CAD ventures!
Author: Hans Henrik Thodberg (c). Published 18 Feb 2012