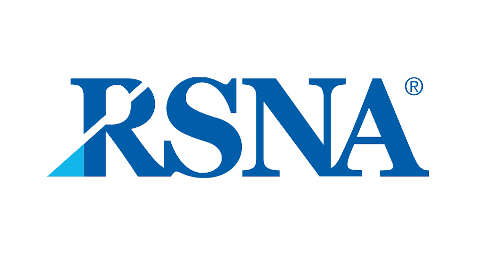
RSNA challenge boosts automated bone age ratings
RNSA (The Radiological Society of North America) organized a machine learning challenge in bone age rating in 2017. This article reviews the challenge and explains its crucial role in boosting performance of BoneXpert in terms of bone age ratings.
On August 5, 2017, RSNA published 12611 hand X-rays with associated bone age ratings, and challenged the machine learning community to develop algorithms for automated bone age rating. 260 teams signed up. For the final test, RSNA released 200 X-rays without rating, and 48 teams submitted bone age predictions. One of several radiologists had rated the 12611 cases. RSNA obtained six independent ratings for the 200 test cases, and the average of these six bone age ratings was defined as the reference, and the Mean Absolute Deviation (MAD) from the reference was used as the measure accuracy for selecting the best algorithms. The top-five algorithms achieved MAD 4.3-4.5 months, and as there was no statistically significant difference in performance between these five, RSNA elected these as winners of the Challenge, and a paper on these five algorithms was written (in Radiology).
Human rating vs. machine learning
Visiana participated with an algorithm similar to version 2 of BoneXpert, but retrained on the RSNA data, and obtained MAD 4.5, ranking number 4.
The basis of the Challenge is that the true bone age rating of an X-ray is the average rating by very many humans.
The first thing one can ask is: how accurate is a single human in hitting the true rating? The answer is MAD = 6.5 months.
The second thing to ask is: how close is the reference rating of 200 test cases to the true rating? The answer is MAD = 2.6 months.
Finally: how close is a new human rating to the reference? The answer is MAD 7.0 months.
Now, the fact that the winning algorithms achieved MAD 4.3-4.5 months wrt the reference is very remarkable. This is so much better than a single human can do, and it relates directly to the hot question: Will AI replace radiologists? – the answer seems to be, that for bone age rating, yes indeed!
It is nice that when one trains a machine learning method on images with a single rating, performed by many raters, the method will learn a good approximation to the true rating. What happens is that during training, the rater variability is averaged out, and the machine ends up being more accurate than a human rater.
BoneXpert improvements after RSNA
After the Challenge, Visiana developed BoneXpert version 3 and released it in September 2019. This embodies three improvements. Firstly, it locates the bones more accurate. Secondly, it finds more bones, namely also finger 2 and 4 and the carpals, so that in total 28 bones are analysed. Thirdly, it uses a record-high number of training examples: 14036 from RSNA, 8250 from Tübingen, 1642 normal cases from Europe and USA, plus about 6000 extra normal cases at the low and high ends of the bone age scale – in total approx. 30000 cases. In contrast, version 2 used less than 2000 cases, so version 3 was in a much better position to learn the intricacies of bone age rating.
Version 3 obtains MAD 4.1 months on the RSNA benchmark test set (and RMSE = 0.45 y), so it outperforms the 47 other algorithms (although it is still not significantly better than the five winners, statistically). The above plots show the performance.
One can derive that the average rating of four humans also gives MAD = 4.1 months wrt the reference, and one can also derive, that the accuracy of version 3 wrt the true rating is MAD 3.2 months.
Impact of RSNA challenge
The RSNA Challenge advanced automated bone age rating in three important ways. Firstly, the main prerequisite for the good performance among the winners was the very large number of training examples provided. Secondly, the Challenge lead to a conceptual clarification of bone age rating, by demonstrating that the average of six ratings reduces the human rater variability and exposes the impressive performance of machine learning. Thirdly, the Challenge established a benchmark for future research.
Performing well on the RSNA benchmark is a necessary, but not sufficient, condition for being clinically useful. The following other aspects of BoneXpert are crucial:
- It is a CE-marked medical device and a supported product.
- It has usability, as proven by the numerous sites who pay per analysis, despite being not reimbursed.
- More than 20 publications have reported validation studies of BoneXpert, addressing specific disorders and populations, presenting reference curves, and validating wrt adult height prediction.
- BoneXpert incorporates an automated method for rejecting dubious bones, or even rejecting the entire image. This is necessary for the use as an autonomous method.
- The algorithm gives visual feedback about which bones were analysed, and exactly how they were located. This “explanation” gives the user confidence in the system, and allows going into detail with a particular case, if desired.
The take-home message for clinical practice is the following: State-of-the-art of bone age rating used to be a careful manual rating by an expert. This has now changed: a machine can do clearly better. So, if a clinic wants to use the best practice, there seems to be only two options
- One can ask four human raters to read each image, and take the average
- One can use BoneXpert
Using a single manual rating is no longer the best practice.